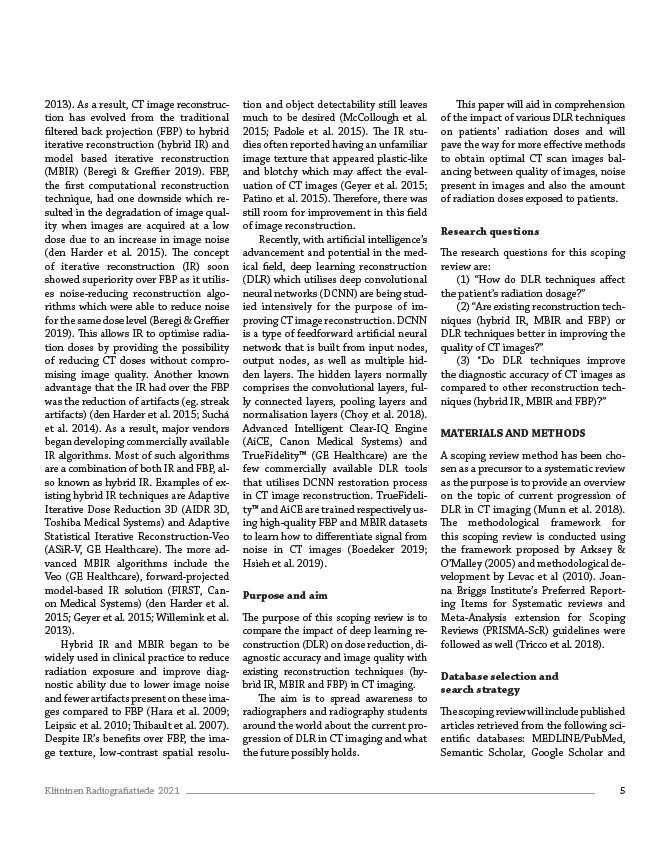
2013). As a result, CT image reconstruc-tion
has evolved from the traditional
filtered back projection (FBP) to hybrid
iterative reconstruction (hybrid IR) and
model based iterative reconstruction
(MBIR) (Beregi & Greffier 2019). FBP,
the first computational reconstruction
technique, had one downside which re-sulted
in the degradation of image qual-ity
when images are acquired at a low
dose due to an increase in image noise
(den Harder et al. 2015). The concept
of iterative reconstruction (IR) soon
showed superiority over FBP as it utilis-es
noise-reducing reconstruction algo-rithms
which were able to reduce noise
for the same dose level (Beregi & Greffier
2019). This allows IR to optimise radia-tion
doses by providing the possibility
of reducing CT doses without compro-mising
image quality. Another known
advantage that the IR had over the FBP
was the reduction of artifacts (eg. streak
artifacts) (den Harder et al. 2015; Suchá
et al. 2014). As a result, major vendors
began developing commercially available
IR algorithms. Most of such algorithms
are a combination of both IR and FBP, al-so
known as hybrid IR. Examples of ex-isting
hybrid IR techniques are Adaptive
Iterative Dose Reduction 3D (AIDR 3D,
Toshiba Medical Systems) and Adaptive
Statistical Iterative Reconstruction-Veo
(ASiR-V, GE Healthcare). The more ad-vanced
MBIR algorithms include the
Veo (GE Healthcare), forward-projected
model-based IR solution (FIRST, Can-on
Medical Systems) (den Harder et al.
2015; Geyer et al. 2015; Willemink et al.
2013).
Hybrid IR and MBIR began to be
widely used in clinical practice to reduce
radiation exposure and improve diag-nostic
ability due to lower image noise
and fewer artifacts present on these ima-
ges compared to FBP (Hara et al. 2009;
Leipsic et al. 2010; Thibault et al. 2007).
Despite IR’s benefits over FBP, the ima-
ge texture, low-contrast spatial resolu-tion
and object detectability still leaves
much to be desired (McCollough et al.
2015; Padole et al. 2015). The IR stu-
dies often reported having an unfamiliar
image texture that appeared plastic-like
and blotchy which may affect the eval-uation
of CT images (Geyer et al. 2015;
Patino et al. 2015). Therefore, there was
still room for improvement in this field
of image reconstruction.
Recently, with artificial intelligence’s
advancement and potential in the med-ical
field, deep learning reconstruction
(DLR) which utilises deep convolutional
neural networks (DCNN) are being stud-ied
intensively for the purpose of im-proving
CT image reconstruction. DCNN
is a type of feedforward artificial neural
network that is built from input nodes,
output nodes, as well as multiple hid-den
layers. The hidden layers normally
comprises the convolutional layers, ful-ly
connected layers, pooling layers and
normalisation layers (Choy et al. 2018).
Advanced Intelligent Clear-IQ Engine
(AiCE, Canon Medical Systems) and
TrueFidelity™ (GE Healthcare) are the
few commercially available DLR tools
that utilises DCNN restoration process
in CT image reconstruction. TrueFideli-ty
™ and AiCE are trained respectively us-ing
high-quality FBP and MBIR datasets
to learn how to differentiate signal from
noise in CT images (Boedeker 2019;
Hsieh et al. 2019).
Purpose and aim
The purpose of this scoping review is to
compare the impact of deep learning re-construction
(DLR) on dose reduction, di-agnostic
accuracy and image quality with
existing reconstruction techniques (hy-brid
IR, MBIR and FBP) in CT imaging.
The aim is to spread awareness to
radiographers and radiography students
around the world about the current pro-gression
of DLR in CT imaging and what
the future possibly holds.
This paper will aid in comprehension
of the impact of various DLR techniques
on patients’ radiation doses and will
pave the way for more effective methods
to obtain optimal CT scan images bal-ancing
between quality of images, noise
present in images and also the amount
of radiation doses exposed to patients.
Research questions
The research questions for this scoping
review are:
(1) “How do DLR techniques affect
the patient’s radiation dosage?”
(2) “Are existing reconstruction tech-niques
(hybrid IR, MBIR and FBP) or
DLR techniques better in improving the
quality of CT images?”
(3) “Do DLR techniques improve
the diagnostic accuracy of CT images as
compared to other reconstruction tech-niques
(hybrid IR, MBIR and FBP)?”
MATERIALS AND METHODS
A scoping review method has been cho-sen
as a precursor to a systematic review
as the purpose is to provide an overview
on the topic of current progression of
DLR in CT imaging (Munn et al. 2018).
The methodological framework for
this scoping review is conducted using
the framework proposed by Arksey &
O’Malley (2005) and methodological de-velopment
by Levac et al (2010). Joan-na
Briggs Institute’s Preferred Report-ing
Items for Systematic reviews and
Meta-Analysis extension for Scoping
Reviews (PRISMA-ScR) guidelines were
followed as well (Tricco et al. 2018).
Database selection and
search strategy
The scoping review will include published
articles retrieved from the following sci-entific
databases: MEDLINE/PubMed,
Semantic Scholar, Google Scholar and
Kliininen Radiografiatiede 2021 5