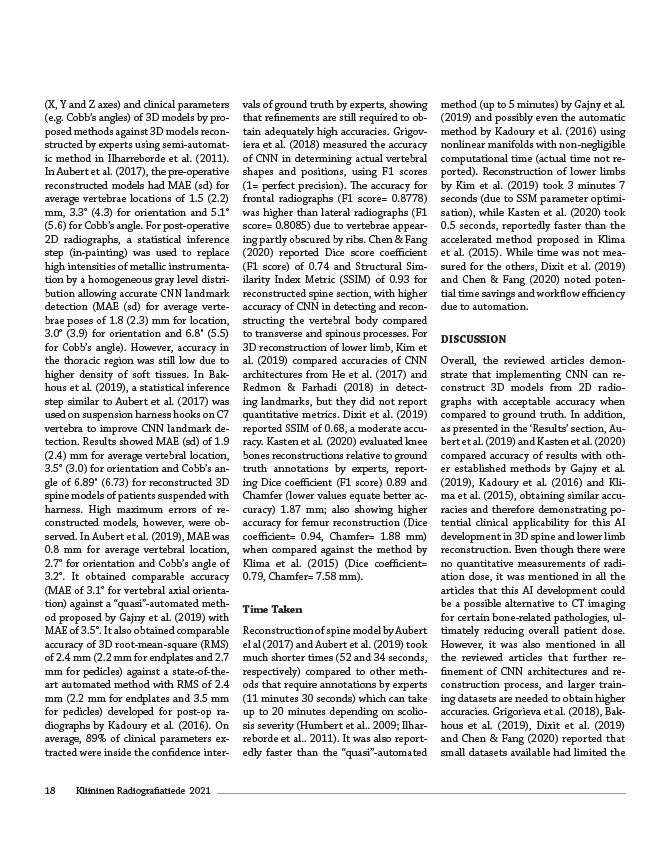
(X, Y and Z axes) and clinical parameters
(e.g. Cobb’s angles) of 3D models by pro-posed
methods against 3D models recon-structed
by experts using semi-automat-ic
method in Ilharreborde et al. (2011).
In Aubert et al. (2017), the pre-operative
reconstructed models had MAE (sd) for
average vertebrae locations of 1.5 (2.2)
mm, 3.3° (4.3) for orientation and 5.1°
(5.6) for Cobb’s angle. For post-operative
2D radiographs, a statistical inference
step (in-painting) was used to replace
high intensities of metallic instrumenta-tion
by a homogeneous gray level distri-bution
allowing accurate CNN landmark
detection (MAE (sd) for average verte-brae
poses of 1.8 (2.3) mm for location,
3.0° (3.9) for orientation and 6.8° (5.5)
for Cobb’s angle). However, accuracy in
the thoracic region was still low due to
higher density of soft tissues. In Bak-hous
et al. (2019), a statistical inference
step similar to Aubert et al. (2017) was
used on suspension harness hooks on C7
vertebra to improve CNN landmark de-tection.
Results showed MAE (sd) of 1.9
(2.4) mm for average vertebral location,
3.5° (3.0) for orientation and Cobb’s an-gle
of 6.89° (6.73) for reconstructed 3D
spine models of patients suspended with
harness. High maximum errors of re-constructed
models, however, were ob-served.
In Aubert et al. (2019), MAE was
0.8 mm for average vertebral location,
2.7° for orientation and Cobb’s angle of
3.2°. It obtained comparable accuracy
(MAE of 3.1° for vertebral axial orienta-tion)
against a “quasi”-automated meth-od
proposed by Gajny et al. (2019) with
MAE of 3.5°. It also obtained comparable
accuracy of 3D root-mean-square (RMS)
of 2.4 mm (2.2 mm for endplates and 2.7
mm for pedicles) against a state-of-the-art
automated method with RMS of 2.4
mm (2.2 mm for endplates and 3.5 mm
for pedicles) developed for post-op ra-diographs
by Kadoury et al. (2016). On
average, 89% of clinical parameters ex-tracted
were inside the confidence inter-vals
18 Kliininen Radiografiatiede 2021
of ground truth by experts, showing
that refinements are still required to ob-tain
adequately high accuracies. Grigov-iera
et al. (2018) measured the accuracy
of CNN in determining actual vertebral
shapes and positions, using F1 scores
(1= perfect precision). The accuracy for
frontal radiographs (F1 score= 0.8778)
was higher than lateral radiographs (F1
score= 0.8085) due to vertebrae appear-ing
partly obscured by ribs. Chen & Fang
(2020) reported Dice score coefficient
(F1 score) of 0.74 and Structural Sim-ilarity
Index Metric (SSIM) of 0.93 for
reconstructed spine section, with higher
accuracy of CNN in detecting and recon-structing
the vertebral body compared
to transverse and spinous processes. For
3D reconstruction of lower limb, Kim et
al. (2019) compared accuracies of CNN
architectures from He et al. (2017) and
Redmon & Farhadi (2018) in detect-ing
landmarks, but they did not report
quantitative metrics. Dixit et al. (2019)
reported SSIM of 0.68, a moderate accu-racy.
Kasten et al. (2020) evaluated knee
bones reconstructions relative to ground
truth annotations by experts, report-ing
Dice coefficient (F1 score) 0.89 and
Chamfer (lower values equate better ac-curacy)
1.87 mm; also showing higher
accuracy for femur reconstruction (Dice
coefficient= 0.94, Chamfer= 1.88 mm)
when compared against the method by
Klima et al. (2015) (Dice coefficient=
0.79, Chamfer= 7.58 mm).
Time Taken
Reconstruction of spine model by Aubert
el al (2017) and Aubert et al. (2019) took
much shorter times (52 and 34 seconds,
respectively) compared to other meth-ods
that require annotations by experts
(11 minutes 30 seconds) which can take
up to 20 minutes depending on scolio-sis
severity (Humbert et al.. 2009; Ilhar-reborde
et al.. 2011). It was also report-edly
faster than the “quasi”-automated
method (up to 5 minutes) by Gajny et al.
(2019) and possibly even the automatic
method by Kadoury et al. (2016) using
nonlinear manifolds with non-negligible
computational time (actual time not re-ported).
Reconstruction of lower limbs
by Kim et al. (2019) took 3 minutes 7
seconds (due to SSM parameter optimi-sation),
while Kasten et al. (2020) took
0.5 seconds, reportedly faster than the
accelerated method proposed in Klima
et al. (2015). While time was not mea-sured
for the others, Dixit et al. (2019)
and Chen & Fang (2020) noted poten-tial
time savings and workflow efficiency
due to automation.
DISCUSSION
Overall, the reviewed articles demon-strate
that implementing CNN can re-construct
3D models from 2D radio-graphs
with acceptable accuracy when
compared to ground truth. In addition,
as presented in the ‘Results’ section, Au-bert
et al. (2019) and Kasten et al. (2020)
compared accuracy of results with oth-er
established methods by Gajny et al.
(2019), Kadoury et al. (2016) and Kli-ma
et al. (2015), obtaining similar accu-racies
and therefore demonstrating po-tential
clinical applicability for this AI
development in 3D spine and lower limb
reconstruction. Even though there were
no quantitative measurements of radi-ation
dose, it was mentioned in all the
articles that this AI development could
be a possible alternative to CT imaging
for certain bone-related pathologies, ul-timately
reducing overall patient dose.
However, it was also mentioned in all
the reviewed articles that further re-finement
of CNN architectures and re-construction
process, and larger train-ing
datasets are needed to obtain higher
accuracies. Grigorieva et al. (2018), Bak-hous
et al. (2019), Dixit et al. (2019)
and Chen & Fang (2020) reported that
small datasets available had limited the