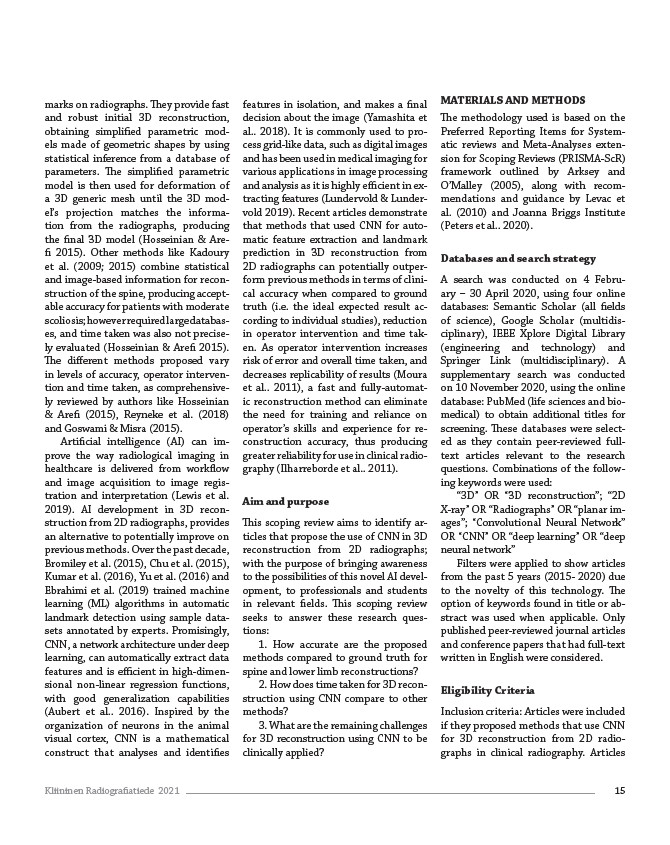
marks on radiographs. They provide fast
and robust initial 3D reconstruction,
obtaining simplified parametric mod-els
made of geometric shapes by using
statistical inference from a database of
parameters. The simplified parametric
model is then used for deformation of
a 3D generic mesh until the 3D mod-el’s
projection matches the informa-tion
from the radiographs, producing
the final 3D model (Hosseinian & Are-fi
2015). Other methods like Kadoury
et al. (2009; 2015) combine statistical
and image-based information for recon-
struction of the spine, producing accept-able
accuracy for patients with moderate
scoliosis; however required large databas-es,
and time taken was also not precise-ly
evaluated (Hosseinian & Arefi 2015).
The different methods proposed vary
in levels of accuracy, operator interven-tion
and time taken, as comprehensive-ly
reviewed by authors like Hosseinian
& Arefi (2015), Reyneke et al. (2018)
and Goswami & Misra (2015).
Artificial intelligence (AI) can im-prove
the way radiological imaging in
healthcare is delivered from workflow
and image acquisition to image regis-tration
and interpretation (Lewis et al.
2019). AI development in 3D recon-struction
from 2D radiographs, provides
an alternative to potentially improve on
previous methods. Over the past decade,
Bromiley et al. (2015), Chu et al. (2015),
Kumar et al. (2016), Yu et al. (2016) and
Ebrahimi et al. (2019) trained machine
learning (ML) algorithms in automatic
landmark detection using sample data-sets
annotated by experts. Promisingly,
CNN, a network architecture under deep
learning, can automatically extract data
features and is efficient in high-dimen-sional
non-linear regression functions,
with good generalization capabilities
(Aubert et al.. 2016). Inspired by the
organization of neurons in the animal
visual cortex, CNN is a mathematical
construct that analyses and identifies
features in isolation, and makes a final
decision about the image (Yamashita et
al.. 2018). It is commonly used to pro-cess
grid-like data, such as digital images
and has been used in medical imaging for
various applications in image processing
and analysis as it is highly efficient in ex-tracting
features (Lundervold & Lunder-vold
2019). Recent articles demonstrate
that methods that used CNN for auto-matic
feature extraction and landmark
prediction in 3D reconstruction from
2D radiographs can potentially outper-form
previous methods in terms of clini-
cal accuracy when compared to ground
truth (i.e. the ideal expected result ac-cording
to individual studies), reduction
in operator intervention and time tak-en.
As operator intervention increases
risk of error and overall time taken, and
decreases replicability of results (Moura
et al.. 2011), a fast and fully-automat-ic
reconstruction method can eliminate
the need for training and reliance on
operator’s skills and experience for re-construction
accuracy, thus producing
greater reliability for use in clinical radio-
graphy (Ilharreborde et al.. 2011).
Aim and purpose
This scoping review aims to identify ar-ticles
that propose the use of CNN in 3D
reconstruction from 2D radiographs;
with the purpose of bringing awareness
to the possibilities of this novel AI devel-opment,
to professionals and students
in relevant fields. This scoping review
seeks to answer these research ques-tions:
1. How accurate are the proposed
methods compared to ground truth for
spine and lower limb reconstructions?
2. How does time taken for 3D recon-struction
using CNN compare to other
methods?
3. What are the remaining challenges
for 3D reconstruction using CNN to be
clinically applied?
MATERIALS AND METHODS
The methodology used is based on the
Preferred Reporting Items for System-atic
reviews and Meta-Analyses exten-sion
for Scoping Reviews (PRISMA-ScR)
framework outlined by Arksey and
O’Malley (2005), along with recom-mendations
and guidance by Levac et
al. (2010) and Joanna Briggs Institute
(Peters et al.. 2020).
Databases and search strategy
A search was conducted on 4 Febru-ary
– 30 April 2020, using four online
databases: Semantic Scholar (all fields
of science), Google Scholar (multidis-ciplinary),
IEEE Xplore Digital Library
(engineering and technology) and
Springer Link (multidisciplinary). A
supplementary search was conducted
on 10 November 2020, using the online
database: PubMed (life sciences and bio-medical)
to obtain additional titles for
screening. These databases were select-ed
as they contain peer-reviewed full-text
articles relevant to the research
questions. Combinations of the follow-ing
keywords were used:
“3D” OR “3D reconstruction”; “2D
X-ray” OR “Radiographs” OR “planar im-ages”;
“Convolutional Neural Network”
OR “CNN” OR “deep learning” OR “deep
neural network”
Filters were applied to show articles
from the past 5 years (2015- 2020) due
to the novelty of this technology. The
option of keywords found in title or ab-stract
was used when applicable. Only
published peer-reviewed journal articles
and conference papers that had full-text
written in English were considered.
Eligibility Criteria
Inclusion criteria: Articles were included
if they proposed methods that use CNN
for 3D reconstruction from 2D radio-graphs
in clinical radiography. Articles
Kliininen Radiografiatiede 2021 15